Ecology and Evolution of Infectious Disease
Pathogens are a ubiquitous component of ecological communities, where they strongly impact numerous aspects of host ecology and evolution. Understanding these ecological and evolutionary dynamics is made urgent by the emergence of new diseases and a need to understand the role of biotic interactions in the dynamics of natural ecosystems. Projects in the lab focus on a range of important questions in disease evolutionary ecology, and do so by combining mathematical models with empirical studies of anther-smut disease (Microbotryum) on wild carnations.
​
Anther-smut is a model system for disease ecology because its biology and transmission have strong analogies to vector-borne and sexually transmitted diseases in animals and humans. It is easily detected in nature by the dark brown spores that take the place of pollen on the anthers, and prevalence estimates are straightforward since the fungus sterilizes but does not kill the host. Moreover, demographic studies, manipulative field experiments, and controlled inoculation studies are much easier in plants than animals.
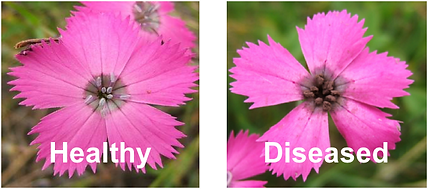
Evolution of age-dependent resistance
Juvenile susceptibility is wide-spread among plants, wildlife, and humans, and plays an essential role in disease dynamics. For example, many of the diseases that have shaped the course of human history, such as measles and smallpox are primarily ‘childhood diseases.’ In Dianthus pavonius seedlings are ten times more susceptible to the disease than adults, that this high level of juvenile susceptibility has allowed disease to persist at high prevalence within populations. Yet we still lack a basic evolutionary explanation for why juvenile hosts retain such high susceptibility. We are using a combination of mathematical modeling, field studies, and experimental evolution to understand the costs, benefits, underlying genetics and numerical feedbacks that drive the evolution of age-specific resistance. This work is funded by a joint NSF-NERC program on 'Bridging Ecology and Evolution" with the Ashby lab at Bath University.
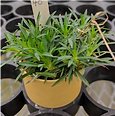
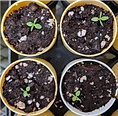

Susceptibility of adult and seedling D. pavonius plants

Feedbacks play a role in the evolution of resistance onset.
Evolution of Resistance Correlations
A fundamental question in disease biology is how the genetic structure of a host population affects disease transmission. When we consider that hosts are bombarded not by one, but by multiple pathogen species (some of them novel!), the correlation of resistance mechanisms emerges as a key feature of a population’s resistance structure. However, we lack a theoretical framework for understanding how such resistance correlations arise and how they affect the invasion potential of foreign pathogens. This project is part of a collaborative NIH-EEID funded grant with the Hood Lab at Amherst College.
​
​
Of particular interest is the joint evolution of specific and general forms resistances that have been described for numerous plant species. General forms of resistance are more likely to exhibit positive correlations in resistance across a broad range of foreign pathogens than specific resistance mechanisms that have co-evolved to recognize only the local endemic pathogen strains. Our initial results show that specific and general resistance genes evolve differently in tandem than when modeled separately, and importantly can impact the magnitude of the resistance correlations between endemic and foreign pathogens.

Effect of the cost of resistance specific R gene on the slope of the resistance correlation between endemic and foreign pathogens for full and half sib families.
Ecology and evolution of transmission mode
Evolution of new transmission modes can play an essential role in disease emergence, and pose serious challenges for disease control (e.g. sexual transmission of zika virus). However, concerns about evolution in emerging infectious diseases has largely focused on increases in virulence and we know much less about the ecological factors driving the evolution of transmission mode.
Anther-smut disease is maintained in nature through two different modes: frequency-dependent vector-transmission to adult flowering plants and density-dependent aerial-transmission to highly susceptible seedlings. Current research is focused on determining how variation in host density and age-structure affects the success of transmission through these two different routes. This work is part of a collaborative NIH-EEID funded project with Janis Antonovics, Michael Hood and Mike Boots.
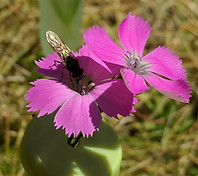
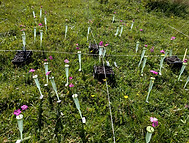
We use arrays of cut flowers in tubes to manipulate density and study spatial patterns of spore deposition through vector and aerial transmission routes
Disease and the evolution of separate sexes
Sex-specific differences in disease exposure have been widely observed throughout the animal and plant kingdoms, yet, whether these differences in disease risk play a role in the incipient evolution of separate sexes (dioecy) from hermaphroditism has been largely overlooked. Dianthus pavonius has a gynodioecious mating system composed of hermaphrodites and female plants. Female flowers have significantly lower levels of spore deposition than hermaphrodite flowers. The mechanism for this difference in exposure appears to be a combination of floral closing behavior in female flowers and strong pollinator preferences for hermaphrodites. Currently we are interested in the effect of disease on hermaphrodite investment into male and female function. Does high disease prevalence select for increased investment in male function in hermaphrodites? We are also interested in the genetics of sex in Dianthus (thought to be cyto-nuclear) and how this affects response to selection from disease.
